GANplifying event samples
31 May 2022
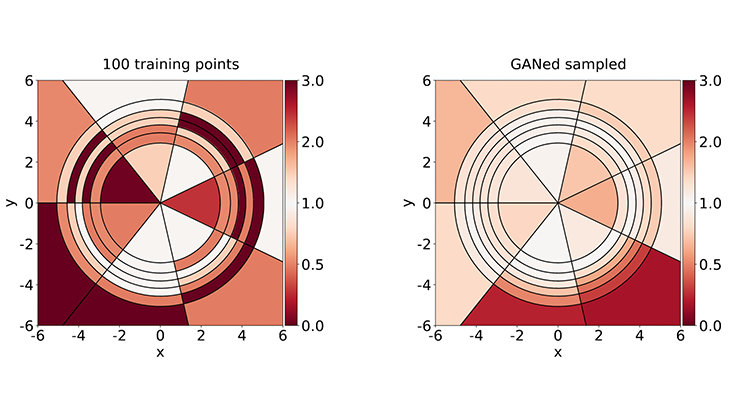
Photo: SciPost (CC BY 4.0)
How the use of generative models in particle physics can improve accuracy
Monte Carlo simulation plays an extremely important role in particle physics. However, these complex and accurate simulations take a significant amount of time. Generative machine learning models gained initial publicity for creating realistic looking "deep-fake" images of persons, but were since demonstrated to be a promising approach for speeding up physics simulations. However, one major roadblock for the practical use of generative models in physics was the question of whether the data generated by the model results in a more accurate description compared to the data used to train the model. This paper investigates this question using a series of small-scale examples and manages to show that generative models can, in fact, result in improved accuracy and are therefore a viable simulation tool.
Publication
Anja Butter, Sascha Diefenbacher, Gregor Kasieczka, Benjamin Nachman, Tilman Plehn: GANplifying event samples, SciPost Phys. 10, 139 (2021).
For his contribution to the publication, Sascha Diefenbacher received a Quantum Universe Best Paper Award in 2022.