Foundation models in particle physicsUsing the power behind Chat-GPT for physics
30 April 2025
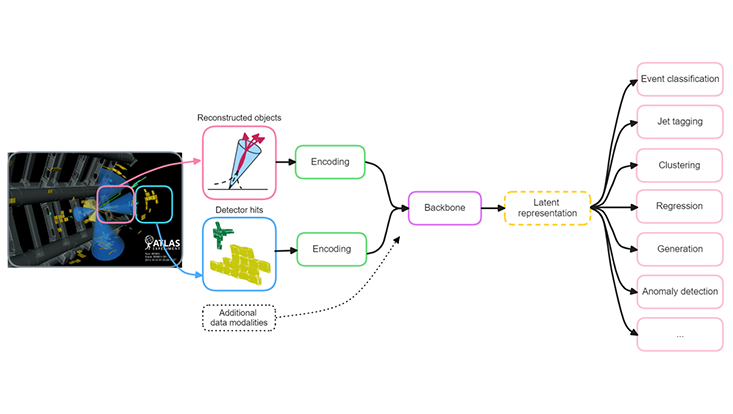
Photo: Joschka Birk
Chat-GPT is great at coming up with birthday card greetings, summarizing complicated texts, or brainstorming ideas for the name of your new dog. But can it do physics? In a recent paper, researchers of the cluster of Excellence Quantum Universe explored the idea behind models like Chat-GPT and applied it to particle physics.
Large language models like Chat-GPT belong to a class of Artificial intelligence models called foundation models. The reason for that name is that they are first pre-trained to learn the general structure of some large dataset. This knowledge then serves as a foundation for further specializations, known as fine-tuning. This means that a model is trained once and for all on some large dataset, and then re-used for a wide variety of specific tasks.
Since foundation models have revolutionized natural language processing, it is natural to ask whether they could also boost physics research. One promising aspect of developing foundation models for particle physics in particular, is that particle physics experiments generate large amounts of data that could be used for pre-training. A well-trained foundation model could boost the achievable performance on specialized tasks requiring expensive simulated data.
This study uses the original GPT architecture as a basis for the model. The approach required the scientists to develop an additional model able to learn how to transform particle physics data into a format the GPT architecture understands. This project specifically focuses on so-called jets, sprays of particles resulting from certain particle decays in the detector. The goal was to design the model such that it performs two common tasks in particle physics: simulating jets (generation) and distinguishing different types of jets from each other (classification). The new model is called OmniJet-α; “omni” because it can do several different things, “jet” because it is developed for jet physics, and “α” because it is a first prototype version.
The idea was to first pre-train the model to generate jets, with the expectation that the model would utilize its knowledge of what jets in general are supposed to look like in the fine-tuning step, where it was asked to classify different jets. For the development of the model, the researchers used a simulated dataset and focused on two types of jets: jets originating from light quarks or gluons, and jets originating from top quarks that decay hadronically. The first type is very common at the Large Hadron Collider (LHC), and the second type is very interesting in part due to the top quark’s strong connection to the Higgs boson.
Once trained on the generation task, the model is able to generate new jets. Figure 1 shows the mass of the generated jets (solid line), compared to the mass of the jets it was trained on (shaded area). The agreement is very good.
Figure 1. Distributions of jet mass for jets from the training data (shaded) and generated jets (solid line). The mass distribution of the generated jets matches the jets from the training data. The figure shows both the low-mass peak of the quark/gluon jets, and a peak at the top quark mass around 175 GeV. (copyright: Joschka Birk et al., CC BY 4.0)
The big question was whether knowing how to generate jets would give the model an advantage in the classification task. To examine this, the researchers adjusted the model architecture such that the model output became a classification score: is this jet more likely to be a quark/gluon jet, or a top jet? To simulate the case with very little labeled data, the scientists tested this fine-tuning step on varying amounts of jets, from only 100 up to 2 million. The test led to an extraordinary result: in order to achieve the same performance that a non-pre-trained model would reach with 1 million jets, the pre-trained model only needs 1000. That is 1000 times less! Figure 2 shows the comparison between the fine-tuned model and the model that was trained from scratch. The y-axis indicates accuracy, which is the fraction of correct classifications. The x-axis shows the number of training events that were used for the training in the classification step.
Figure 2. Accuracy, or in other words, fraction of correct classifier outputs, for a classifier making use of the pre-trained model (fine-tuning, green) and a classifier that didn’t have access to the pre-trained model (from scratch, red). The different classification training data sizes are listed on the x-axis. The solid line corresponds to the mean of five different trainings, and the shaded region to the standard deviation. The figure shows that the fine-tuned model is able to make use of the head start it receives from the pre-training, and that it achieves the same performance as the model trained from scratch with much less training data. (copyright: Joschka Birk et al., CC BY 4.0)
OmniJet-α was the first foundation model for particle physics able to perform two different tasks: generation and classification. Since this model was published, the scientists have also shown that the generative part of it can directly be used on CMS Open Data, and that the model also can be used to generate calorimeter showers, a type of particle data that is very different from the jets the model was developed for.
The exploration of foundation models for particle physics is now in full swing, in Hamburg as well as at other universities and institutes all over the world, and it is very exciting to be part of such an active field. Will researchers be able to build models that are as powerful for physics as they have been for natural language and computer vision? This pioneering work is a first step on the way, demonstrating not only how to harness the power of vast unlabeled datasets, but also that it is possible to create foundation models that once pre-trained, can be specialized to several different tasks.
Best Paper Award 2025
The authors of the study received a Best Paper Award (category Postdoc) by the Cluster of Excellence for their pioneering work.